Navigating the Ethics of Technology and AI in Aid Diversion Prevention
- Katja Hemmerich
- Jul 23, 2023
- 6 min read
Updated: Aug 15, 2024
23 July 2023
By Katja Hemmerich
This week the WFP Executive Board receives a management update on its operations in Ethiopia, which were suspended in June when widespread diversion of food aid was discovered. Cindy McCain, the new Executive Director of WFP has indicated that the organization will revamp the way commodities and beneficiaries are tracked and traced
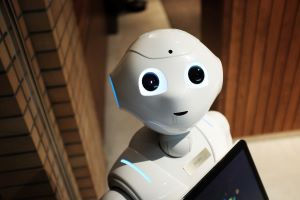
through enhanced use of technology. WFP’s draft Management Plan for 2024-2026 goes on to propose spending $23.7 million over two years on new monitoring systems.
Our spotlight explores different uses of technology and artificial intelligence (AI) to prevent aid diversion and corruption, and the benefits and risks involved. We then suggest some questions for those participating in WFP’s management briefing to help make informed decisions on mitigating those risks.
The most immediate benefit of technology in preventing and identifying corruption is that it can automate processes and allow them to be monitored, while making it much harder for individuals to manipulate the process for their own gain. Beyond the anti-corruption benefits, automated processes are often more efficient and quicker. A positive example of this is the use of biometric data and technology by WFP and UNHCR to streamline food and cash transfers in refugee programming in Syria and Uganda, for instance.
In Syria, through WFP and UNHCR's partnerships with supermarkets and banks, iris scans are used to authenticate the identity of recipients and to allow them to access cash or food assistance without vouchers, PINs, or documentation. This has been perceived as positive also by recipients, one of whom explained:
"It is an accurate, easy and fast process. [By means of] eye-scanning [there is] no need to show some documents to prove my identity or wait several hours to receive the assistance. I do not need to go back to my home empty-handed either because I forgot the correct password. All I need is to stare at the machine and all of the information appears on the screen." – B. Paragi & A. Altamimi, ‘Caring control or controlling care? Double bind facilitated by biometrics between UNHCR and Syrian refugees in Jordan’ Society and Economy (2022), pg. 220
Artificial intelligence, and its ability to analyze huge volumes of data from multiple different sources provides even more possibilities to use data to prevent, or identify patterns of, potential corruption. Primarily national governments have been experimenting with such tools to identify fraud in public procurement or tax evasion, or to allow citizens to better hold public officials accountable. For instance, in Brazil “Rosie the Robot” sifts through the expenses reported by Congressional officials and flags those it deems suspicious, for instance if purchases indicate the Congress member was in two different locations on the same day and time. Rosie then tweets her findings, inviting citizens to corroborate or dismiss the suspicions and asking congress members to justify themselves. Rosie’s crowdsourcing for validation is typical of many ‘bottom up’ approaches to anti-corruption. (P. Aarvik, Artificial Intelligence - A Promising Anti-Corruption Tool in Development Settings, Anti-Corruption Resource Centre, 2019)
India, by contrast, has taken a ‘top down approach’ in its use of artificial intelligence to detect tax evasion. The Finance Ministry’s ‘Project Insight’ scours citizen data on purchases and expenditures, including on social media, to determine spending patterns and compares that with their tax filings. While Project Insight has resulted in less tax evasion, it has also been widely criticized for violating citizens’ privacy rights. (P. Aarvik, 2019)
The questions about privacy rights and who has a right to use various types of citizens' data is a key risk for any such anti-corruption endeavor, including by international organizations. Data confidentiality concerns have been raised in connection with WFP and UNHCR’s use of biometric data to register refugees in Syria, where refugees alleged that the data was used to monitor their movements without their knowledge. When refugees were confronted with the fact that staff of international organizations knew when and where they crossed borders, it led to suspicion about what data was being shared with immigration authorities in the region or elsewhere, raising protection concerns and trust issues vis-a-vis international organizations (Paragi & Altamimi, 2022).
One strategy to mitigate this risk is to explain to beneficiaries how their data is going to be used once collected to allow for their informed consent when data is collected. On paper this seems easy to manage, and UNHCR and WFP have policies for this, but in practice it is fraught with challenges. As researchers found when studying the Syria example, many refugees did not remember the data issues being explained or being asked to consent. This may have reflected weaknesses in how WFP or UNHCR staff communicated the information, but it likely also reflected that many refugees had little or no understanding of these issues, and most importantly, were desperate to get assistance. Without registering, which they understood required sharing of their biometric data, they would have been refused assistance and the possibly the right to remain in Jordan. (Paragi & Altamimi, 2022).
A novel approach to managing data and protection risks could therefore be to spread the risk beyond affected populations to other actors. Brazil’s Comptroller General has developed an application to estimate the risk of corrupt behavior of its civil servants by suing AI to analyze data on how the person was recruited, criminal records, business and shareholder relationships and political affiliations among other things. The algorithm was trained using a large dataset on convictions of civil servants (P. Aarvik, 2019). Since the problems in Ethiopia were reportedly related to various government and military personnel involved in food distribution, consideration could be given to using AI to monitor risks of corruption amongst their personnel along with collecting biometric data on aid recipients. This would mean the data privacy risks are not just borne by affected populations.
Beyond sharing of data, other anti-corruption efforts that use artificial intelligence have demonstrated potential risks related to decision-making. Because the AI algorithms can be quite complex and the machine learning process that takes place is not easily explained, allowing AI to take decisions on its own can be fraught with risk. Research has shown that algorithms can reinforce and learn the same biases of the humans that create them. This is precisely the reason that Amazon dismantled its own AI recruiting tool when it discovered that by ‘learning’ from previous recruitments, the tool was prioritizing applications from male candidates (P. Aarvik, 2019).
Decisions on recruitments, whether to charge someone with tax evasion or whether someone can access food aid significantly impact the life of that person and their families. Consequently, affected people should have the right to have those decisions explained. This ‘right to explanation’ is included in the European General Data Protection Regulation (GDPR), but is not necessarily systematically included in national legislation everywhere or in regulations of international organizations.
An additional, and perhaps more effective, way of mitigating this risk is also using AI and technology to assist humans as decision-makers, rather than letting them make decisions on their own. Many successful uses of tech in anti-corruption simply flag where humans should be paying more attention in their decision-making. (Odilla, F. 'Bots against corruption: Exploring the benefits and limitations of AI-based anti-corruption technology', Crime, Law and Social Change (2023))
In short, technology and artificial intelligence have significant potential to prevent and identify corruption in assistance programming. But they also come with risks, which need to be considered a mitigated. As researchers from the Max Planck Institute for Human Development summarized:
"Top–down use of AI-ACT [anti-corruption tools] can consolidate power structures and thereby pose new corruption risks. Bottom–up use of AI-ACT has the potential to provide unprecedented means for the citizenry to keep their government and bureaucratic officials in check." – Köbis, N., Starke, C. & Rahwan, I., 'The promise and perils of using artificial intelligence to fight corruption', Nature Machine Intelligence, (2022)
With this in mind, we suggest some questions for those participating in the Ethiopia management review and discussions on improved monitoring systems at WFP:
What risks have been identified with the new monitoring systems and how will they be mitigated?
Will the affected populations alone be subject to collection and analysis of their personal data? Are there means to monitor activities of other stakeholders as well?
Will technology determine when corruption exists, or will it simply assist humans in making decisions? Will those affected by decisions regarding corruption or receipt of assistance be able to challenge those decisions?
Key Meetings of UN Governance Mechanisms this Week